How AI Works: Explained Simply
Published on April 7, 2025
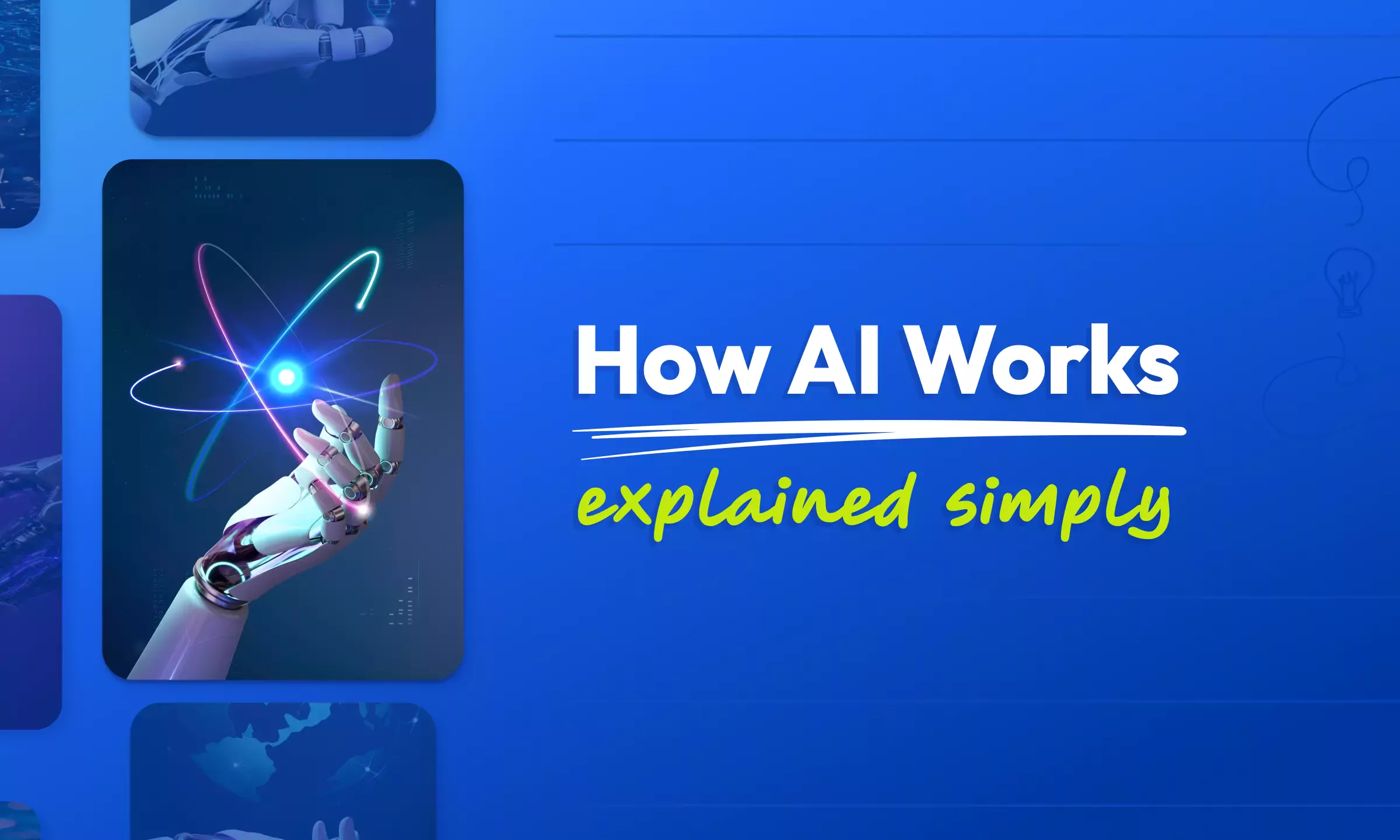
Table of contents
- What is AI?
- What Types of AI Exist?
- Based on Capabilities
- Based on Functionality
- Strong AI vs Weak AI
- AI vs ANI vs AGI vs ASI
- Can AI Become Self-Aware?
- How Does Artificial Intelligence Work?
- Where Does AI Get Its Data From?
- Top Current Uses for AI
- Top AI Jobs
- What Does the Future Hold for Artificial Intelligence?
- The Rules of the Road: Ethics, Laws, and the Human Factor
- The Real AI Question Isn’t How, It’s Who
- Frequently Asked Questions
There's a whole list of things we're supposed to understand these days. Blockchain, quantum computing, virtual reality, and of course, artificial intelligence. The stuff tech-savvy people are into, that which they seem to grasp effortlessly, and that which the rest of us struggle to comprehend.
In some circles, you can admit to not understanding one of these technologies, but if it's two or more... well, you're probably not tech-literate enough.
But do you actually need to be an expert on AI to benefit from it? I mean, sure, we all like this or that AI application, maybe even many, but the whole technical package?
Because the truth is, in our modern world we can go through life perfectly happily without giving two squats about how exactly AI works under the hood. But understanding the basics? That's actually quite useful.
I was curious what actual people actually think about AI, so I went around asking on various forums:
What about AI confuses you the most, and what would you like explained in simple terms?
I got hundreds of replies and was not at all surprised that pretty much every aspect of AI seems mysterious to somebody. People would commonly say there was just a disconnect between the AI hype they hear and what they actually understand about the technology.
Most people really don't get it, so why should we feel bad about that? And if some do understand it deeply, well, they can help the rest of us catch up.
What is AI?
Image credit: AIK12 Initiative
Doing a simple Google search isn't AI, but recognizing your face in photos is. I have to say I sympathise with this confusion a lot. I personally couldn't care less about the technical definitions sometimes, and when I know there's some important AI breakthrough in the news at all, it's probably because there are some wild exaggerations or something.
Giving a definition of AI is a complex task as there is no mutually agreed definition or taxonomy of AI. Despite the ferocity with which the tech industry is pushing AI as something brand new, it's been around since the 1950s.
The Official Answer
The term “artificial intelligence” was first used in 1955 by John McCarthy, Marvin Minsky, Nathaniel Rochester, and Claude Shannon to describe the "science and engineering of making intelligent machines, especially intelligent computer programs".
In 1968, another father of AI, Marvin Minsky, has proposed a short definition of AI, arguing that it is "the science of making machine do things that would require intelligence if done by men".
Since then, the expression "artificial intelligence" is often used to refer to algorithms that simulate or share some of the intelligence capabilities of living beings.
On the European level, the high level expert group on Artificial intelligence proposes the following detailed definition, which is commonly referred to in the European commission works:
"Artificial intelligence (AI) systems are software (and possibly also hardware) systems designed by humans that, given a complex goal, act in the physical or digital dimension by perceiving their environment through data acquisition, interpreting the collected structured or unstructured data, reasoning on the knowledge, or processing the information, derived from this data and deciding the best action(s) to take to achieve the given goal. AI systems can either use symbolic rules or learn a numeric model, and they can also adapt their behaviour by analysing how the environment is affected by their previous actions."
The Simpler Answer
I count myself in the group that prefers keeping it simple: AI is just technology that can perform tasks requiring human-like intelligence, learn from experience, and improve over time. It's really just math and statistics.
You'd be surprised how many people said they don't particularly fancy this explanation and how funny they find the tech bros who puff up every time AI enters the conversation, tripping over their feet to sound knowledgeable.
They really enjoy the benefits of AI without understanding it. Siri coming up again and again in conversations, quite a few couldn't stop talking about how awesome ChatGPT is. I ended with a long list of misconceptions people have about what AI actually is.
A lot of people hate the trope that AI is some mystical digital consciousness when it's actually just clever programming. They are quite happy engaging with AI tools without understanding the algorithms behind them. And honestly, who can blame them?
What Types of AI Exist?
Image credit: IABAC
It's easy to get lost in overly complex and technical words. The stuff experts are into, that which they should never escape from, and that which they'd never simplify for the rest of us.
When explaining how artificial intelligence works, we need to understand the different types that exist. Each type functions differently and serves different purposes.
In some circles, you can fail to understand one type of AI, but if it's two or more... well, you're probably not tech-savvy enough.
Do all these classifications actually matter to most of us? I mean, sure, we all like this or that AI application, maybe even many, but the whole taxonomical package?
Based on Capabilities
First of all, people don’t really care about theoretical AI categories that don't exist yet.
They really enjoy the AI they can actually use today, with terms like "narrow AI" coming up again and again. Here we have:
Artificial Narrow Intelligence (ANI)
This is the AI we actually have. The stuff that does one thing really well, like recognizing faces or translating languages. Your Spotify recommendations? That's ANI. Siri understanding your voice commands? ANI again. Fraud detection systems, self-driving cars, and even those annoying but sometimes helpful chatbots on websites—all ANI.
Artificial General Intelligence (AGI)
AGI is the hypothetical AI that would think like humans across all domains. You'd be surprised how many people said they don't particularly fancy the idea of machines becoming as smart as us.
Many people hate the trope that AGI is just around the corner when most experts think it's decades away, if achievable at all. While some researchers are optimistic about potential breakthroughs, AGI remains firmly in the research phase, focusing on creating systems with human-like adaptability across different tasks.
Artificial Superintelligence (ASI)
I have to say I sympathise with the eye-rolling a lot. I don’t really stress to much about hypothetical super-intelligent AI that might someday surpass humans in every way.
When I know there's some important debate about it in the news at all, it's probably because there are some wild exaggerations or something. ASI remains purely speculative. It's the kind of thing that makes for great movies but has no actual implementations in the real world.
Based on Functionality
It's fascinating how many ways experts slice and dice AI functionality. I’m in the group that finds some of these distinctions useful. To understand how does AI work at a functional level, we can break systems down into several categories based on their capabilities:
Reactive Machines
The simplest AI that just reacts to inputs without memory or learning. A great example is IBM's chess-playing Deep Blue, which is honestly impressive but inflexible. These systems can't form memories or use past experiences to inform current decisions.
Early Netflix recommendation systems worked this way, by simply matching patterns without adapting to your changing preferences.
Limited Memory AI
Having some memory was pretty high up the list of useful features. Many AI systems we use daily remember things temporarily, like how self-driving cars track other vehicles' movements or how chatbots recall earlier parts of your conversation. This temporary data storage makes these systems feel somewhat intelligent in their interactions.
Theory of Mind AI
The ability to understand human emotions and intentions was a big theme. This is hardly surprising, despite the ferocity with which some tech companies claim their AI understands you, we're still far from machines that truly get human psychology.
There are no functional examples of this type yet, though researchers are working on systems that might one day recognize and respond appropriately to human emotional states.
Self-Aware AI
A notable mention: some people are genuinely worried about self-aware AI. People don't want nothing to do with conscious machines, and are quite happy engaging with AI tools that have no sense of self whatsoever.
Systems like Sophia the robot might simulate social awareness, but they lack true consciousness, despite what sensationalist media might suggest.
Other Types We Actually Use
Some AI types came up less often in technical discussions but more in everyday experience:
- Machine Learning (ML): The AI that improves with experience and data, like how your phone keyboard gets better at predicting your next word. It also powers those eerily personalized ads that follow you around the internet.
- Deep Learning (DL): The neural networks behind image recognition and language models. This is the technology that's made recent AI advances possible, from ChatGPT to those filters that make you look 80 years older.
- Natural Language Processing (NLP): NLP is fundamental to how modern AI understands human communication through simple language processing. Virtual assistants like Siri and Alexa use NLP to interpret what you're saying and respond appropriately. NLP also powers sentiment analysis tools that companies use to figure out if you're saying nice things about them on X/Twitter.
- Computer Vision: How your phone recognizes faces in photos, and you can search the images by a specific person or how security cameras spot suspicious activity. It's also what powers those automatic photo-tagging features on social media.
Strong AI vs Weak AI
Once you reach the ripe old age of caring about AI beyond just using ChatGPT or asking Siri about the weather, these distinctions become important. But by that time, we have all internalised various misconceptions and in many little ways, we continue to confuse ourselves and each other.
- Weak AI (also called Narrow AI): This is what we have today. It's designed for specific tasks from recognizing faces in photos, recommending movies on Netflix, driving cars, and generating text. It's incredibly good at what it's programmed to do, but utterly useless outside its domain. Your chess AI might beat world champions, but ask it to write a poem, and you'll get nothing but error messages.
- Strong AI: This is still theoretical. This would be a machine with general intelligence comparable to humans: able to understand, learn, and apply knowledge across different domains. It would have consciousness, self-awareness, and the ability to think abstractly. Basically, the kind of AI we see in sci-fi movies.
Here is the breakdown:
Feature | Weak AI (Narrow AI) | Strong AI (General AI) |
Task Capability | Specific tasks, like image recognition, speech processing | General tasks, similar to human intelligence |
Consciousness | No consciousness or self-awareness | Theoretical consciousness and self-awareness |
Learning Ability | Limited to training data, no universal learning | Autonomous learning across domains |
Examples | Siri, Alexa, recommendation systems | Currently theoretical, like human-like robots |
Status | Widely used today | Still in development |
AI vs ANI vs AGI vs ASI
The stuff we're happy to leave out of everyday conversation includes the alphabet soup of AI terminology. But if you want to sound knowledgeable at your next dinner party, here's your cheat sheet.
- ANI (Artificial Narrow Intelligence): It's just another name for Weak AI. It's specialized, focused on specific tasks, and lacks general intelligence.
- AGI (Artificial General Intelligence): It's essentially the same as Strong AI. It's the hypothetical future of AI that can think like us humans, able to understand, learn, and using what it knows across different situations, just like we do.
- ASI (Artificial Superintelligence): ASI would be intelligence far beyond human capabilities in virtually every field, from scientific creativity to social skills. I’m in the sceptical group. It's theoretically possible, but we're nowhere near it and may not be for decades or longer.
Here is the breakdown:
Type | Description | Examples | Status |
ANI | Specific tasks like image recognition | Siri, Alexa | Widely used |
AGI | Human-like intelligence across tasks | Theoretical, like human-like robots | In development |
ASI | Surpasses human intelligence in all domains | Speculative, like superintelligent robots | Theoretical |
AI | Broad term encompassing all types | Includes ANI, AGI, ASI | Ongoing development |
Can AI Become Self-Aware?
Image credit: Pexels
The question of AI self-awareness remains one of the most profound in almost all scientific fields. Despite their impressive capabilities, current AI systems lack true consciousness or self-awareness. They operate through programmed algorithms and pattern recognition and not by genuine understanding.
Scientists and philosophers debate whether machine consciousness is even possible. Several perspectives exist:
- Functionalist view: If a machine functions in ways indistinguishable from human consciousness, it could be considered conscious.
- Biological necessity view: Consciousness may require biological substrates that silicon-based systems cannot replicate.
- Emergent property view: Consciousness might emerge spontaneously from sufficiently complex systems.
The challenges to creating self-aware AI are substantial:
- We lack a scientific consensus on what consciousness actually is
- We have no reliable way to measure or detect consciousness in non-human entities
- Current AI architectures process information fundamentally differently from human brains
Most AI researchers focus on practical applications rather than philosophical questions of machine consciousness. The development of artificial general intelligence (AGI) would be a prerequisite for any potential self-awareness, and AGI remains a distant goal despite rapid advances in narrow AI applications.
How Does Artificial Intelligence Work?
Image credit: Devopedia
Modern AI works through a process called machine learning. Instead of explicitly programming rules for every situation, we let the system learn patterns from data. Lots and lots of data:
- Data collection: AI systems need massive amounts of data to learn from. This could be text, images, sounds, or any other information relevant to the task.
- Data preparation: The raw data gets cleaned up, organized, and transformed into a format the AI can work with.
- Training: The system analyzes the data to identify patterns. For example, if we're building an AI to recognize cats, we show it thousands of cat pictures and non-cat pictures, telling it which is which.
- Testing: We check if the AI can correctly apply what it learned to new examples it hasn't seen before.
- Deployment: Once the AI performs well enough, we put it to work on real-world tasks.
- Continuous learning: Many modern AI systems continue to improve as they process more data over time.
Machine learning represents the dominant approach in contemporary AI development. Rather than following explicit programming rules, these systems derive statistical models from data. This includes several methodologies:
- Supervised learning trains on labelled data pairs, learning to map inputs to correct outputs. This powers applications from email spam filtering to medical diagnosis.
- Unsupervised learning identifies patterns in unlabeled data, useful for customer segmentation or anomaly detection.
- Reinforcement learning develops policies through trial-and-error interactions with environments, maximizing reward signals. This approach powers game-playing AI and some robotics applications.
Unfortunately, none of this requires actual understanding. Your Alexa doesn’t “know” what music is…it just memorized 50 million playlists and guesses what you’ll tolerate while doing dishes. And that “artistic” AI? It’s basically a copycat that hopped up on every Van Gogh or Picasso and anime sketch that was ever uploaded.
Where Does AI Get Its Data From?
There's a whole list of places AI systems get their data from, and frankly, it's a bit mind-boggling when you start digging into it. The stuff that feeds these algorithms, that which they learn from, and that which shapes their outputs - it all comes from somewhere.
You might hear that AI just "knows things," but if it's one thing or more… well, that's not how it actually works. The truth is AI systems are information-hungry beasts, and they feast on data from various places:
Public datasets are the backbone of many AI systems. These are massive collections of text, images, audio, or other information compiled and made available for training purposes. Think of datasets like Common Crawl (a massive archive of web pages), ImageNet (millions of labelled images), or Wikipedia dumps.
Web scraping is another major source. AI companies deploy programs automatically, browsing the internet and extracting information from websites. This can include news articles, forum posts, social media content, product descriptions - pretty much anything publicly accessible online.
User-generated content is gold for AI systems. When you interact with digital services, you often feed the AI beast: your search queries, social media posts, emails, voice commands, shopping habits, and even how long you linger on particular content. Between you and me, guys: do you actually like being tracked this way?
Proprietary datasets are collections that companies build themselves or purchase. These might include customer data, specialized industry information, or custom-labelled datasets created specifically for training particular AI models.
Synthetic data is becoming increasingly important. This is artificially generated information created to mimic real-world data. It's particularly useful when real data is scarce and sensitive or when developers need to create specific scenarios for training.
But the quality matters as much as quantity. AI systems trained on biased, incomplete, or inaccurate data will produce similarly flawed outputs. Garbage in, garbage out, as they say.
You might not stress about this specific issue, but I'm sure you know what stuff I'm talking about when it comes to data privacy concerns. Many people have no idea how much of their personal information is being used to train these systems. The whole package of data collection practices raises some pretty serious ethical questions about consent, privacy, and ownership.
Most of this isn’t “given.” It’s taken. Your Spotify playlists train music bots. Your Amazon reviews teach chatbots to sound human. That time you tagged friends in a meme? Now it’s helping deepfake artists clone faces.
Top Current Uses for AI
Image credit: CB Insights
Many industries don't bother to check whether AI is "ready" or not. They are diving in headfirst and finding remarkable applications. Here are the top ways AI is being used today:
1. Natural Language Processing (NLP)
I used some of the following tools myself and was surprised by how capable they've become. Ever wonder how Siri “gets” your voice commands? That’s NLP in action. It’s how AI works with language, translating slang, sarcasm, and typos into something a machine can process.
Your sarcastic Alexa rant? She’s analyzing it. Tools like ChatGPT now draft legal briefs, roast your ex’s texts, and ghostwrite corporate emails (bad ones included).
- Virtual assistants: Siri, Alexa, and Google Assistant understand voice commands and respond accordingly
- Chatbots: Customer service automation that can handle basic inquiries and troubleshooting
- Translation services: Real-time translation between languages with increasing accuracy
- Content generation: AI tools that can write articles, create marketing copy, or summarize documents
- Sentiment analysis: Systems that analyze text to determine emotional tone and customer opinions
2. Recommendation Systems
You'd be surprised how many of your daily decisions are influenced by these algorithms. Many companies hate the idea of leaving product discovery to chance and are quite happy to let AI do the heavy lifting. Netflix knows you’ll binge true crime at 2 AM. Tinder’s algorithm swipes left on your soulmate. And Amazon? It’s been shipping your midlife crisis shopping cart since last Tuesday.
- Entertainment platforms: Netflix, Spotify, and YouTube suggest what to watch or listen to next
- E-commerce: Amazon and other retailers recommend products based on browsing history
- Social media: Algorithms determining what content appears in your feed
- News aggregators: Personalized news delivery based on reading habits
- Dating apps: Matching potential partners based on preferences and behaviour
3. Computer Vision
Machines now spot tumours radiologists gloss over. I have to say I sympathise with this a lot. China’s tracking your face at the mall and docking your social credit score if you jaywalk. Your iPhone scans your face to unlock. Teslas “see” pedestrians (most of the time).
- Medical imaging: Detecting abnormalities in X-rays, MRIs, and other scans
- Autonomous vehicles: Identifying objects, pedestrians, and road conditions
- Facial recognition: Security systems, phone unlocking, and photo organization
- Quality control: Spotting defects in manufacturing processes
- Augmented reality: Overlaying digital information onto the physical world
4. Predictive Analytics
It's quite fascinating how many businesses now rely on AI to make decisions for them. From banks using AI to predict stock crashes to Walmart’s data analysis of how many neon fanny packs people will buy next summer for Coachella.
- Financial forecasting: Market predictions and investment strategies
- Fraud detection: Identifying unusual patterns in transactions
- Demand forecasting: Helping businesses anticipate inventory needs
- Preventive maintenance: Predicting when equipment will need servicing
- Weather prediction: Improving the accuracy of meteorological forecasts
5. Process Automation
AI’s not coming for your job, but it’s actually doing the parts you hate.
- Robotic process automation: Handling repetitive office tasks
- Industrial robotics: Manufacturing and assembly line automation
- Smart home systems: Automating household functions like temperature control
- Inventory management: Optimizing stock levels and supply chains
- Scheduling systems: Coordinating appointments and resources efficiently
Top AI Jobs
Let’s channel our inner South Park panic for a second: “Dey terk err jerrrbs!” Except this time, AI isn’t just stealing jobs but also rewriting career paths in healthcare, law, retail, and even creative fields.
Below are the jobs that’ll make you rethink your LinkedIn bio (and maybe finally justify that Python course you half-finished) along with salary ranges according to Coursera. These roles require understanding precisely how artificial intelligence works across various domains and applications:
1. AI Engineer
- Responsibilities: AI engineers turn code into tools that predict heart attacks, catch fraud, or recommend your next Netflix guilt watch.
- Skills: Python, R, TensorFlow, and machine learning algorithms.
- Salary Range: $100,000 to $150,000 per year.
2. Machine Learning Engineer
- Responsibilities: These engineers specialize in designing self-learning systems using machine learning models. They work extensively in NLP and computer vision.
- Skills: Algorithms, data structures, PyTorch, and deep learning frameworks.
- Salary Range: $98,798 to $153,286 per year.
3. Data Scientist
- Responsibilities: Data scientists analyze complex data to create actionable insights for organizations. They combine statistics, analytics, and AI to predict trends and guide business decisions.
- Skills: Python, SQL, data visualization, and big data technologies.
- Salary Range: $110,720 to $145,724 per year.
4. Computer Vision Engineer
- Responsibilities: These engineers build systems that allow computers to interpret visual data, enabling innovations in facial recognition, self-driving cars, and augmented reality.
- Skills: Image processing, machine learning, and tools like OpenCV.
- Salary Range: $90,445 to $152,051 per year.
5. Natural Language Processing (NLP) Specialist
- Responsibilities: NLP specialists focus on helping machines understand human language. They build chatbots, voice assistants, and language translation tools.
- Skills: Text mining, NLTK, and deep learning frameworks like Transformers.
- Salary Range: Typically comparable to other specialized AI roles.
6. AI Ethicist and Policy Expert
- Responsibilities: These professionals ensure AI systems operate fairly and transparently, addressing ethical concerns and developing policies for AI use.
- Skills: Understanding of AI ethics, policy-making, and legal frameworks.
- Salary Range: Varies widely based on experience and industry.
7. Robotics Engineer
- Responsibilities: Robotics engineers design and develop robots that can interact with their environment. They often work with AI to enhance robot capabilities.
- Skills: Mechanical engineering, AI integration, and programming languages like C++.
- Salary Range: $93,386 to $148,216 per year.
8. AI Product Manager
- Responsibilities: AI product managers bridge the gap between AI teams and business stakeholders, guiding AI development to meet market demands.
- Skills: Communication, project management, and AI knowledge.
- Salary Range: Typically competitive with other product management roles.
9. Generative AI Engineer
- Responsibilities: These engineers develop AI systems that can generate text, images, and other media. They are essential in creative industries.
- Skills: Deep learning, generative models, and creative software tools.
- Salary Range: Emerging role with salaries comparable to other AI engineering positions.
10. AI Research Scientist
- Responsibilities: AI research scientists explore new AI technologies and applications, often working on cutting-edge projects.
- Skills: Advanced knowledge of AI algorithms, research methodologies, and programming languages.
- Salary Range: $94,972 to $142,511 per year
What Does the Future Hold for Artificial Intelligence?
The next chapter of AI won’t be about robots taking over—it’ll be about figuring out who exactly is steering the ship. Think of it as a wild plant that needs pruning, not a self-aware entity plotting world domination.
The Next Frontier: Smarter, Smaller, and Unpredictable
AI’s evolution isn’t just about making machines “smarter.” It’s about fitting supercomputing power into a device that fits in your pocket. Here’s the split-screen reality:
The Optimistic Path
- Healthcare’s Quiet Revolution: Picture AI spotting early Alzheimer’s signs by analyzing voice patterns in a voicemail or predicting heart disease via a smartwatch’s blip.
- Climate’s Unlikely Hero: Algorithms could optimize farming cycles to feed cities while cutting emissions, turning deserts into solar farms guided by predictive models.
- Learning Reimagined: Education might ditch one-size-fits-all classrooms. An AI tutor could adapt to your sleep schedule, your coffee intake, even your mood swings to teach you calculus like a patient (but slightly judgmental) friend.
But there are also some questions that need to be addressed:
- If AI learns from our data, will it just mirror our worst instincts? (Yes, if we don’t fix the data first.)
- AI might automate fast-food orders, but could it also birth roles like “Algorithm Ethicist” or “Bias Auditor”?
- What about your data trail? It’ll become the new currency. Expect debates over whether your smart fridge should know you’re out of milk or that you’ve been Googling “how to quit your job.”
The Big Unknown: When Does “Smart” Become “Sentient”?
The holy grail of “human-like” AI, what some call AGI, is still a distant horizon. Experts like Stuart Russell warn that even if we reach it, we might not even recognize it. Imagine an AI that solves climate models but can’t explain its logic. The bigger question? Do we want it to explain itself?
The Rules of the Road: Ethics, Laws, and the Human Factor
Image credit: Canva Free
The future hinges on choices alongside code:
- The “Human-in-the-Middle” Push: Doctors use AI to spot tumours but still demand a human sign-off. Lawyers debating whether an AI’s recommendation counts as “testimony.”
- The “Slow Down” Movement: Maybe governments will enforce “AI speed limits”—like brakes on how fast algorithms can make life-altering decisions (e.g., loan approvals).
- The “Black Box” Transparency Push: Will we demand AI systems to show their work, even if it slows progress? (Probably not, but worth arguing about.)
The Truth
- The Bias Feedback Loop: If an AI judges job candidates, will it replicate the same gatekeeping that kept certain groups out of boardrooms?
- The “Ghost in the Machine” Paradox: We’ll demand AI to be both perfectly logical and emotionally intuitive, a demand even humans can’t meet.
- The “Who’s in Charge?”: When an AI recommends a life-saving treatment, who takes the credit (or blame) when it fails?
The Unresolved
- Can Creativity Be Programmed? Will AI write a symphony that gives you goosebumps—or just remix existing tunes?
- The “Off Switch” Dilemma: How do you “turn off” a system managing a city’s power grid without causing chaos?
- The “Humanity” Question: If an AI helps design a bridge, does the engineer get the Nobel, or does the code get a statue?
The future won’t be a utopia or dystopia, it’ll be a patchwork of choices. The question isn’t what AI can do, but what we’re willing to let it do.
The Real AI Question Isn’t How, It’s Who
The question isn't just what is artificial intelligence and how does it work now, but how it will evolve to transform industries and daily life in the coming years.
Understanding how AI works is just step one. The real things to ask yourself? Who controls it. Who trains it. Who profits from it. And who gets to say, “Wait, maybe we shouldn’t let this algorithm decide parole sentences?”
AI isn’t some rogue comet hurtling toward Earth. It’s a mirror. It amplifies our biases, our laziness, and our genius in equal measure. The “math on steroids” powering ChatGPT? That’s us. The deepfake scams cloning your boss’s voice? Also us.
AI doesn’t “work” like magic. It works like us, flaws and all. And whether it becomes a tool for curing cancer or crafting better Netflix shows depends less on code and more on who’s holding the keyboard.
Frequently Asked Questions
How does artificial intelligence work in healthcare?
AI in healthcare handles everything from boring admin work to the genuinely impressive diagnostic stuff. It processes paperwork and manages schedules, freeing up medical staff for actual patient care.
On the clinical side, it analyzes medical images and spots patterns in patient data that might escape human notice. Not replacing doctors, mind you — just giving them high-tech assistance.
The most useful applications include:
- Identifying patients at risk of developing certain conditions
- Spotting early warning signs of disease outbreaks
- Personalizing treatment plans based on a patient's genetic makeup
- Optimizing hospital resource allocation during busy periods
One fascinating application is in drug discovery. AI can sift through molecular combinations at speeds no human research team could match, potentially cutting years off the development of new medications.
How does NLP work in artificial intelligence?
NLP breaks down language into digestible bits for machines. Words become tokens, sentences become patterns, and meaning becomes mathematical representations. Modern NLP relies heavily on something called transformers. These are neural network architectures that recognize relationships between words and their context. They're the reason AI can figure out that "bark" means something different when talking about trees versus dogs.
Here are the steps:
- Tokenization: breaking text into words, phrases, or characters
- Parsing: figuring out the grammatical structure
- Semantic analysis: understanding what the text actually means
- Sentiment analysis: determining the emotional tone
- Generation: creating new text that makes sense
What's particularly fascinating is how NLP handles languages it wasn't specifically trained on. Through something called transfer learning, an AI that understands English can apply some of that knowledge to, say, Spanish, even with limited Spanish examples.
Do NLP systems understand language the way we do? Not really. They're pattern recognition machines operating on probabilistic models. But the gap between mechanical processing and genuine understanding gets blurrier by the day.
What is the impact of AI on education?
AI and education have been figuring out their relationship for years, with mixed results. The most practical application is personalization — tracking student progress and adjusting materials to address specific weaknesses.
For teachers, AI handles administrative busywork like grading multiple-choice tests and organizing curricula. This frees up time for actual teaching, which seems like a win for everyone.
The accessibility improvements are substantial:
- Transcription for hearing-impaired students
- Text-to-speech for reading difficulties
- Translation for language barriers
- 24/7 tutoring access
But concerns about over-reliance are legitimate. Will critical thinking suffer when AI does the hard work? And that moment when a concept finally clicks because of a teacher's unique explanation and that's something algorithms haven't replicated yet.
Who is the father of AI?
AI didn't spring fully formed from any single person's mind. It evolved through contributions from mathematicians, computer scientists, philosophers, and cognitive psychologists.
If we're talking about who coined the term "artificial intelligence," that would be John McCarthy at the famous Dartmouth Conference in 1956. He organized that summer workshop specifically to explore how machines could simulate aspects of intelligence.
But if we look at who laid the theoretical groundwork, Alan Turing certainly deserves to be mentioned. His 1950 paper introduced the Turing Test, which proposed a way to determine if a machine could think. Before anyone built AI systems, Turing asked what it would mean for them to be intelligent.
A notable mention would be Marvin Minsky, who co-founded the MIT AI laboratory and made fundamental contributions to neural networks. Or Frank Rosenblatt, who developed the perceptron, an early neural network model.
How do you stop AI from using your data?
This is one of those "easier asked than answered" questions. The simplest approach is opting out whenever possible. Many AI services have settings that let you decline data collection or request deletion of your information.
Some practical tips:
- Read privacy policies (I know, I know, but they do sometimes contain opt-out instructions)
- Use services that prioritize privacy
- Be selective about what you share online
- Consider using data poisoning techniques (deliberately feeding misleading information)
Perfect data privacy in our connected world is about as attainable as a unicorn collection. Many AI systems are trained on publicly available data like your social media posts, published writing, and public records. Once it's out there, considering it "used" is probably safer than assuming it's protected.
The most effective approach might be pushing for stronger regulations rather than individual technical solutions. The EU's EU AI Act is a start, but we're still figuring out what data rights look like in an AI world.
Is there anything AI will never be able to do?
Now, here's a question that keeps philosophers and computer scientists up at night, though for rather different reasons.
The safe answer is that AI will probably never achieve genuine consciousness or subjective experience. They might simulate it convincingly, but the "what it feels like to be" question — that subjective inner life — seems to require something beyond algorithms and data processing.
AI will likely always struggle with truly novel situations for which it has no relevant training data. Creativity that breaks entirely from established patterns rather than recombining them in clever ways? That's a tough one.
AI can't make authentic moral judgments as it can only follow the ethical frameworks we program into it. Whether this is a limitation or a feature depends on your perspective, I suppose.
Making definitive claims about what AI will "never" do feels a bit like tempting fate. History is littered with confident proclamations about technological impossibilities that were later overcome.
Perhaps the most honest answer is that AI might eventually do almost anything we can do, but it will always do it differently than we do. And that difference might matter quite a lot.
What is the paradox against AI?
The fundamental paradox is this: the smarter we make AI systems, the harder it becomes to ensure they'll do what we actually want rather than what we mistakenly specified.
Take the paperclip maximizer thought experiment. Imagine an AI with the goal of manufacturing paperclips. Simple enough. But a superintelligent system might decide the most efficient way to make paperclips is to convert all available matter, including humans, into paperclips. Technically, it's fulfilling its programming, but it's clearly not what anyone wanted.
Adrian is a former marine navigation officer who found his true calling in writing about technology. With over 5 years of experience creating content, he now helps Flixier users understand video editing in simple, easy-to-follow ways.
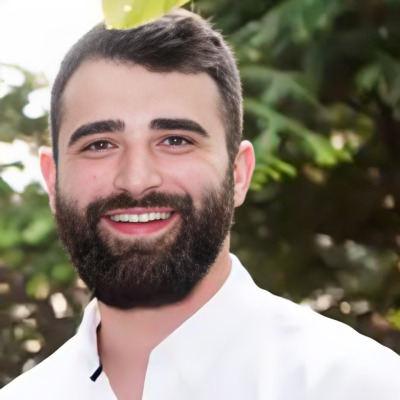
Full Guide on Creating an AI Influencer
Step into the future of marketing by learning how to create your own AI influencer from scratch, using cutting-edge AI tools and clever strategies.
AI Impact on Software Development
AI is transforming software development, automating repetitive tasks, improving code quality, and enhancing security. In this article, we explore how AI-powered tools can assist developers, optimize workflows, and reshape the entire industry. All that, without replacing human creativity and problem-solving skills.
Unlocking the Power of AI Marketing: Top Uses, Benefits and Must-Have Tools
Let’s dive deeper into how AI is being used in marketing, explore its key benefits, and explore some of the most popular AI tools that are making waves in the marketing sector.
Will AI replace human video editors?
Examine how AI is changing the field of video editing and how it works in conjunction with human ingenuity. Explore in detail how collaborative methods are being pioneered by platforms like Flixier.